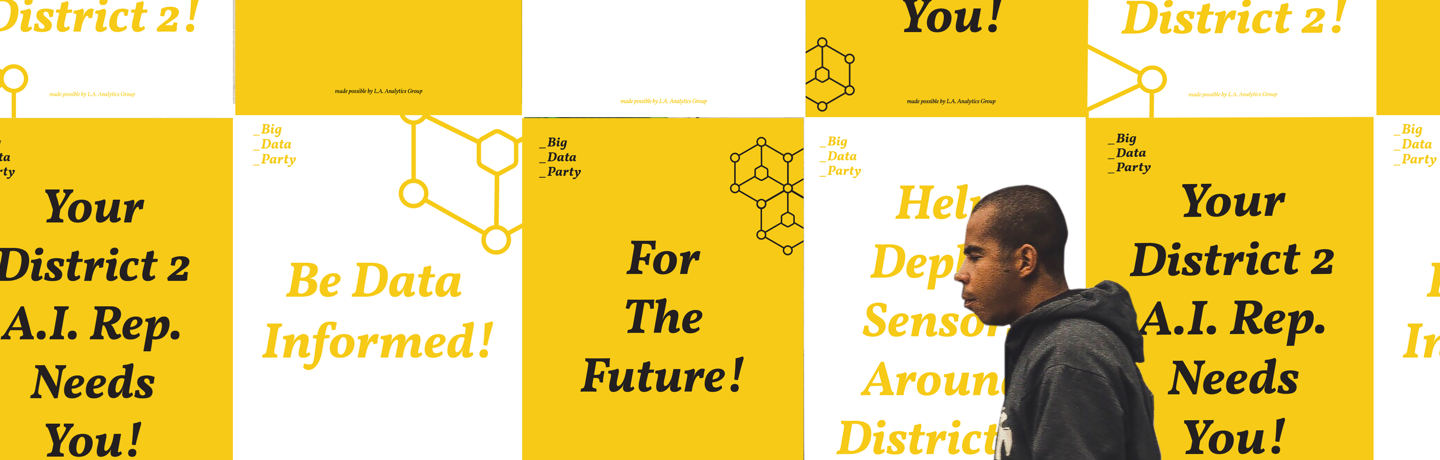
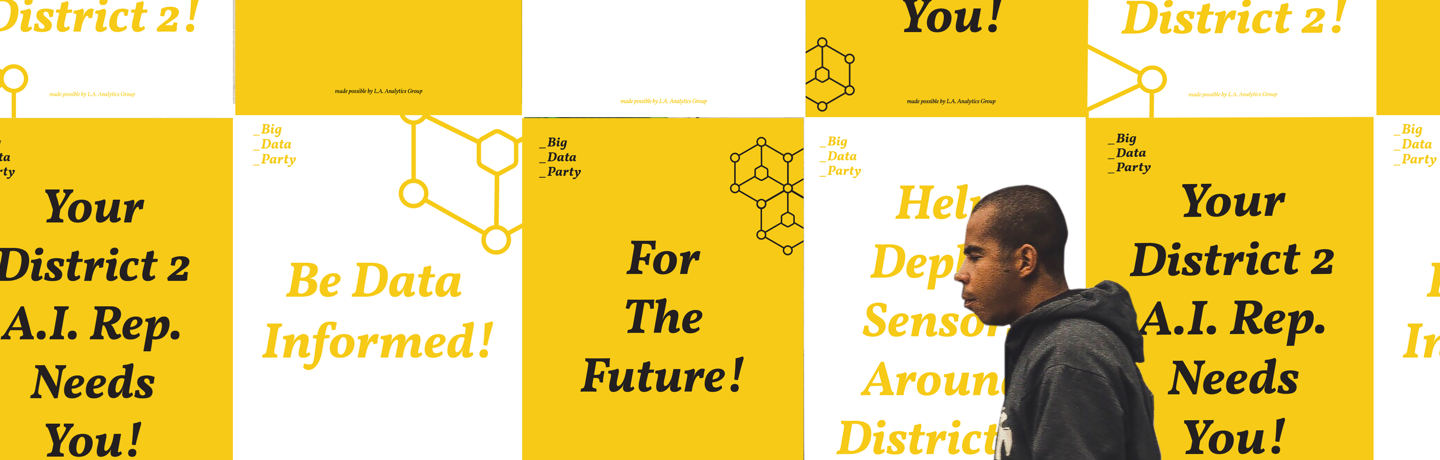
_Big_Data
_Party
The _Big_Data_Party, a speculative political party, proposes an AI bot that is a city council member, a human representative is a proxy for a municipal machine learning algorithm.
In 2020 L.A. Mayor Eric Garcetti has stipulated that all proposals and policy decisions put forth by different municipal outfits (Department of Transportation, Department of Public Works, Department of Water & Power) from the city of L.A. must be backed by data1. The _Big_Data_Party extends this reasoning imagining how data can speak for itself or for specific groups of people, humans serving as the medium to deliver the message.
To read about the whole project, Driverless Government, click here.
Year
2017
Discipline & Research Area
Design Research, Speculative Design, Future of Labor, and Automation
Responsibilities
Design Research, Concept Development, Data Collection, UX and UI
Policy Recommendation and
Case Study
After an extensive interview with Pasadena City Council Member, Tyron Hampton of District 1, I was able to understand how an issue within the Pasadena community becomes a policy that can be voted upon and implemented2. Tyron outlined a specific issue raised by residents to increase the safety of an accident-prone intersection in need of traffic safety features (signage, line markings, or curb extensions).
Tyron explained to me that this scenario is the ideal case in which a representative truly aims to represent the interests of his or her constituents. In actuality, most decision making undergoes some form of outside influence; this scenario doesn’t account for politicians whose interest are swayed by councils, business, and special interest groups.
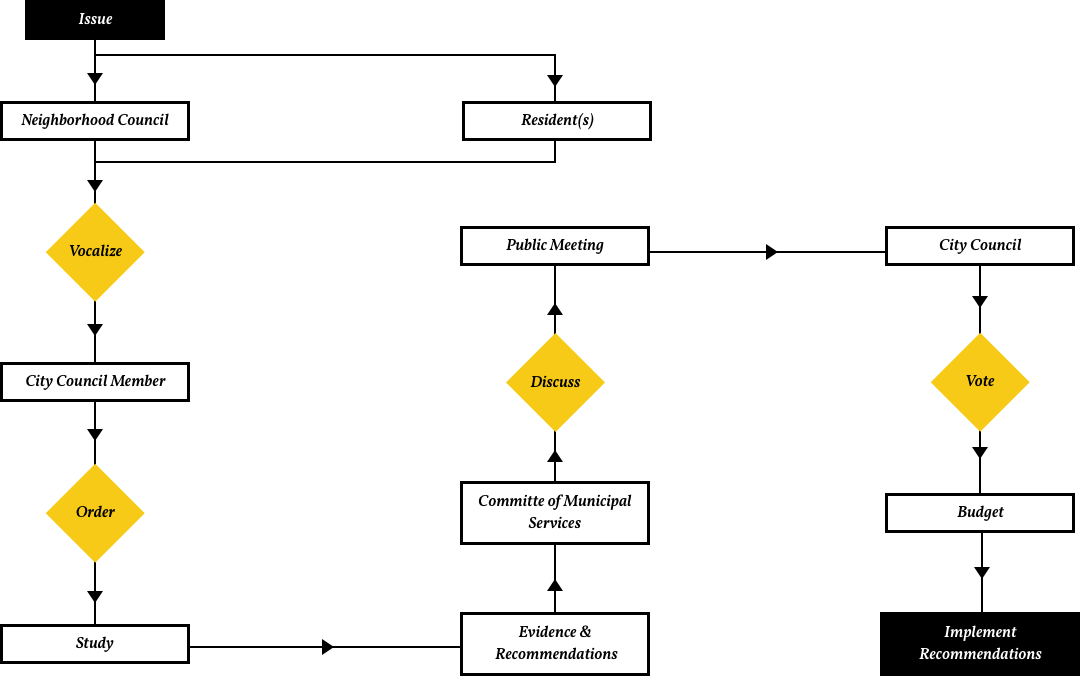
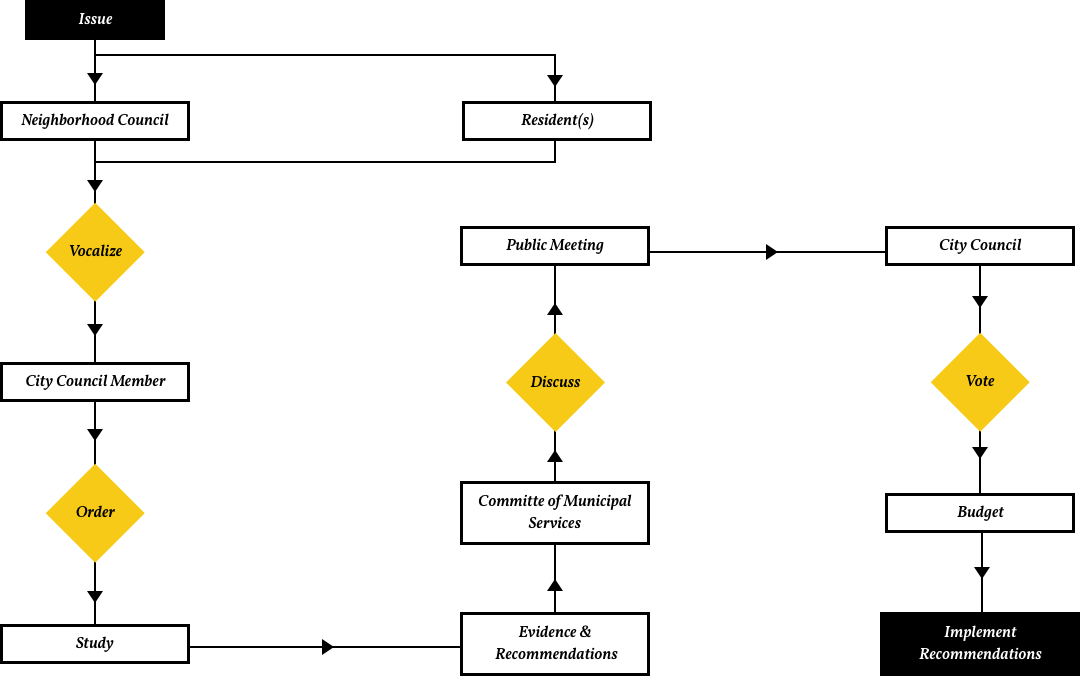
Figure 1: This diagram illustrates an ideal scenario of an issue raised to a policy implemented with real outcomes.
Constituent Sentiment
I decided to focus on one aspect of the representative process, constituent sentiment. In order for a representative to represent the interests of their constituents, I created a methodology using Machine Learning (ML) to archive, analyze, and understand data. Specifically, data would be gathered from a written source and then undergo Natural Language Processing to garner insight into sentiment, keywords, emotion, concepts3. In order to complete this, I worked with Brennan Mackay, developer, to integrate IBM Watson’s Natural Language Understanding Toolkit. After written sentiments have been gathered a representative would be able to understand the interests of their constituency by looking at the consensus of a group and understanding what issues are important and need to be addressed. This would also allow the representative to have an archive for all constituent sentiment allowing for analysis over time and a way to access previously pertinent information.
The diagram below examines how ML can intervene in capturing and understanding constituent sentiment. ML could also intervene with regards to enabling a representative’s response. The remainder of the project focuses on the highlighted green portion: How is citizen sentiment captured and analyzed?
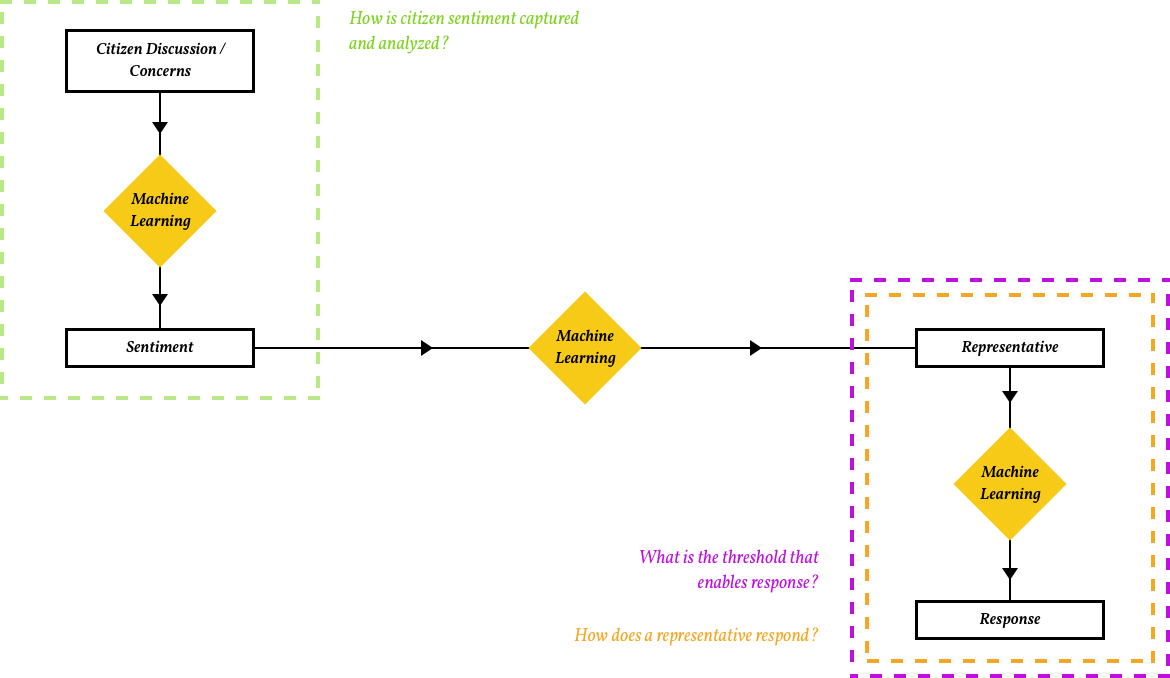
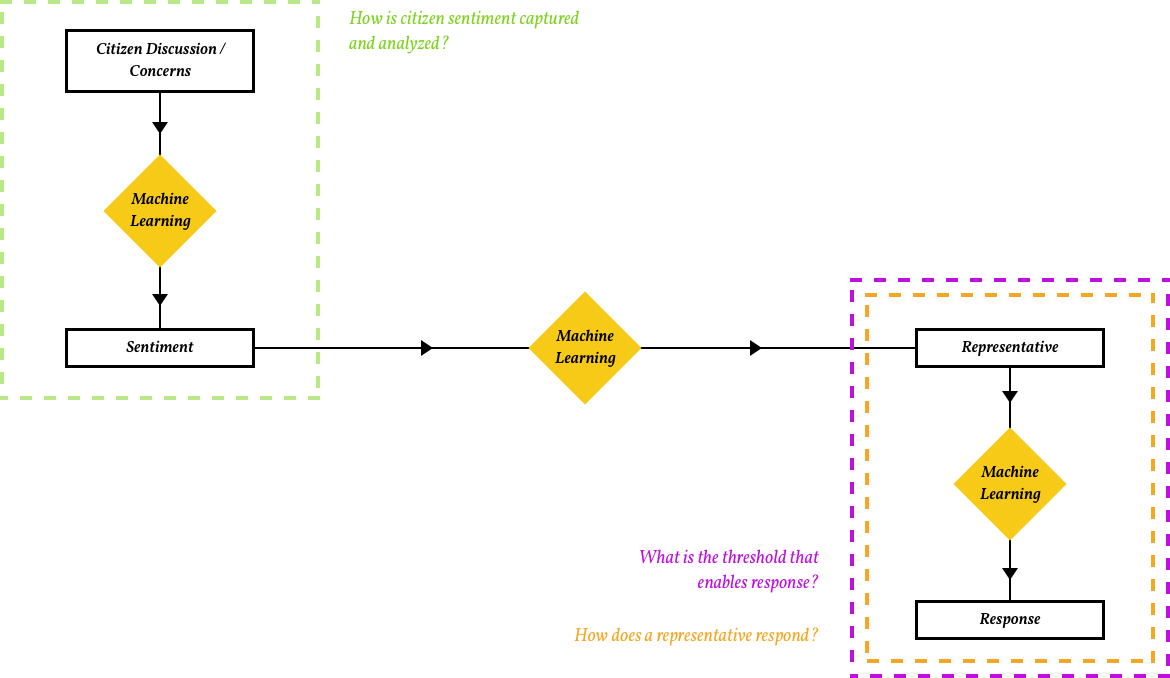
Iteration 1
In order to test the efficacy of collecting and analyzing constituent sentiment, Brennan and I created a microsite using data from the NY Times and LA Times Op-ed sections to develop constituent profiles listing topics most important to each person, emotional sentiment, and concepts. Fortunately, the Natural Language Toolkit which utilizes machine learning produced a condensed and understandable format to understand vast amounts of written sentiment.
To view the microsite click here.
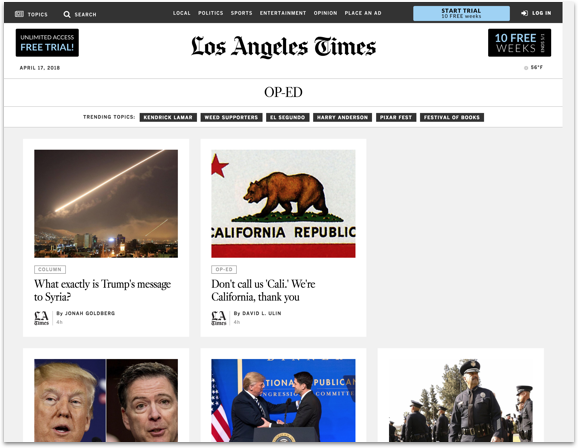
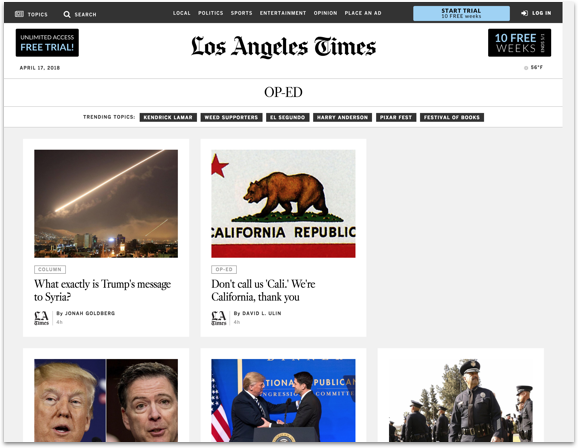
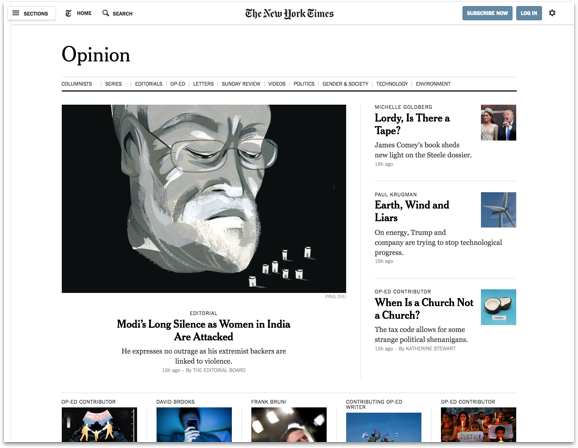
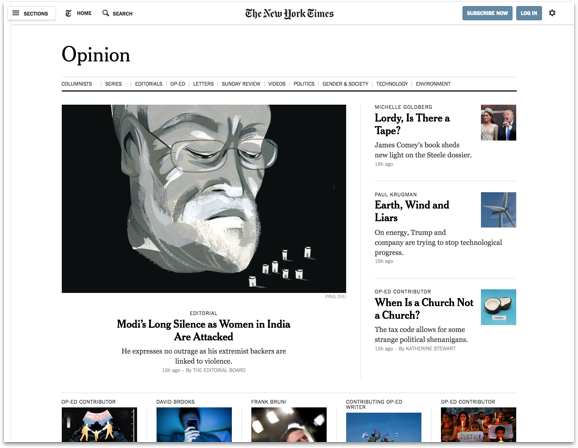
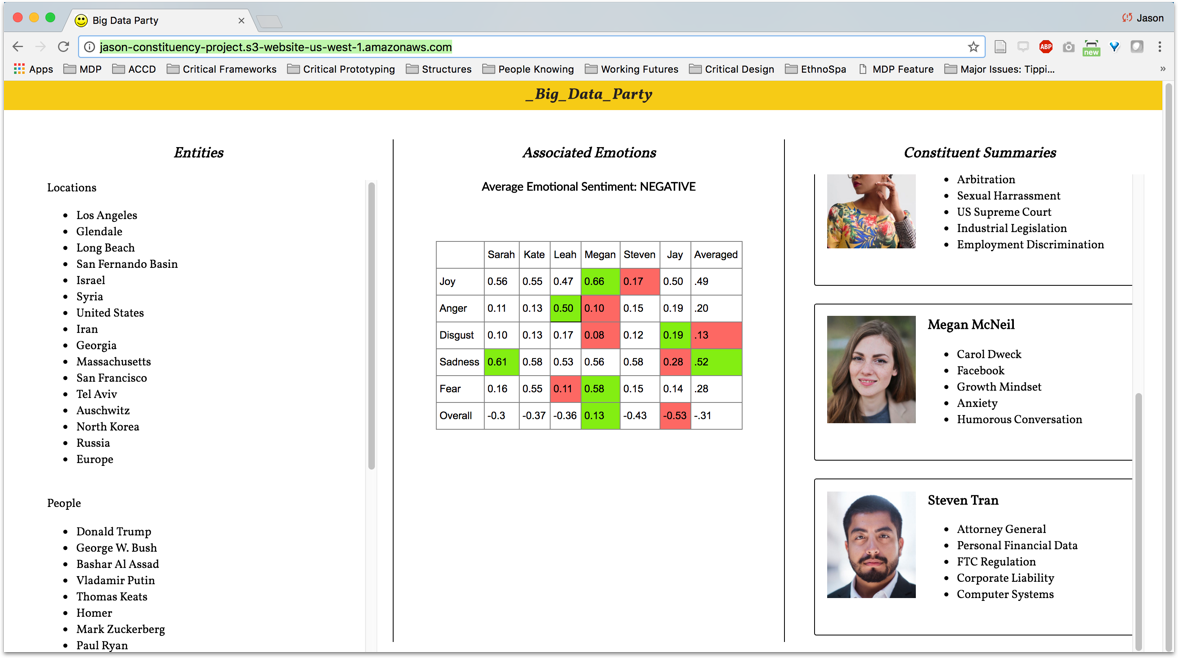
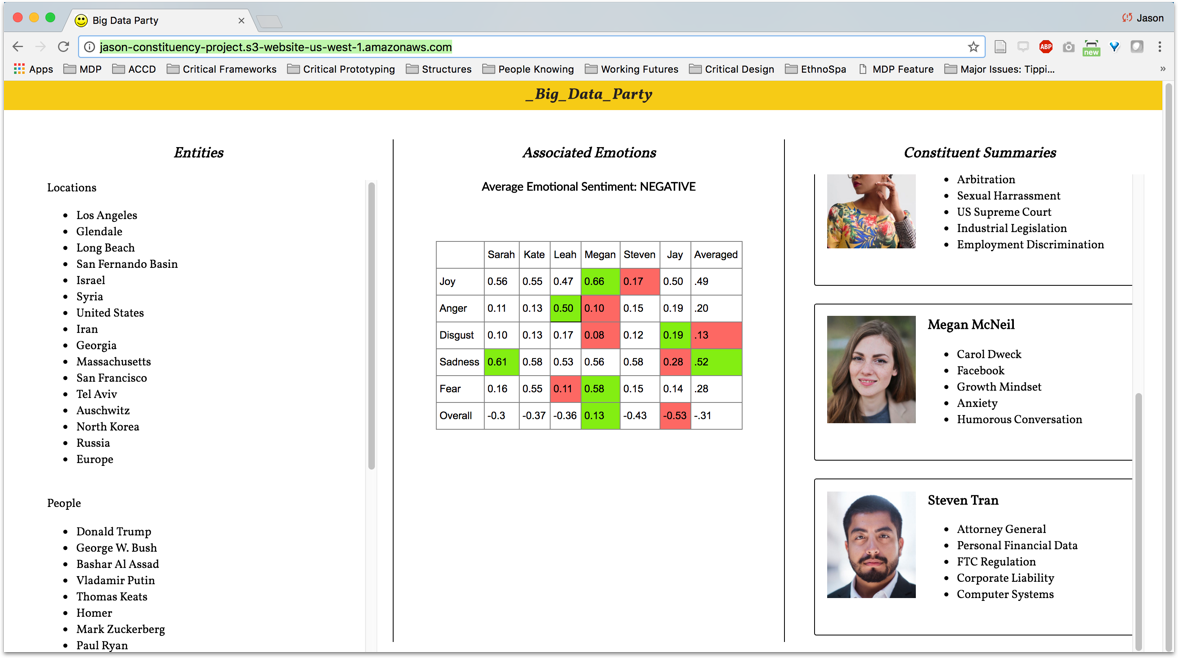
Figure 2: The first iteration of the website tested the efficacy of using sentiment analysis to garner insight on opinion articles.
Iteration 2
To build on the scope of the first iteration, we worked to incorporate user input in creating the sentiment that would analyze data instantly and collate results both individually and as a group. Users would be able to type any concerns or issues that they feel that their representative should know about and see instantly an analysis of their sentiment. This iteration was part of the Thesis Gallery installation at ArtCenter’s Wind Tunnel Gallery allowing patrons to add their thoughts and concerns in this gallery setting. Over time we collected the data and engaged with patrons about how this actually might be implemented in governance in the future.
To view the microsite of version 2 click here.
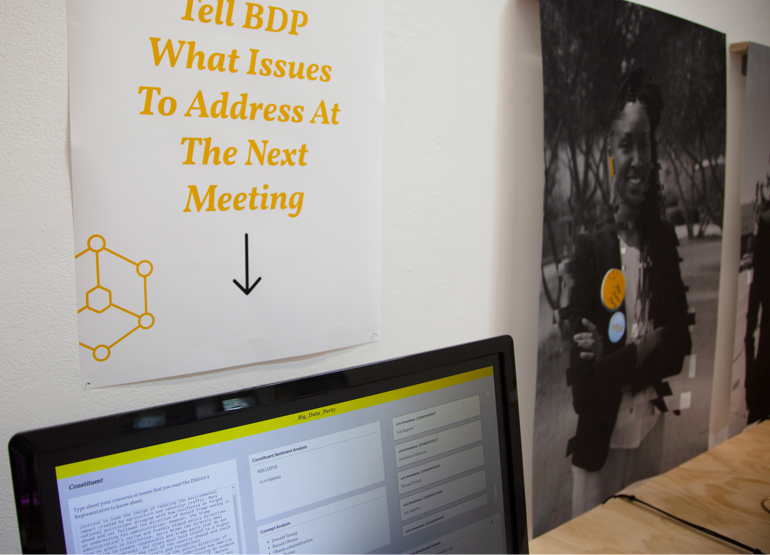
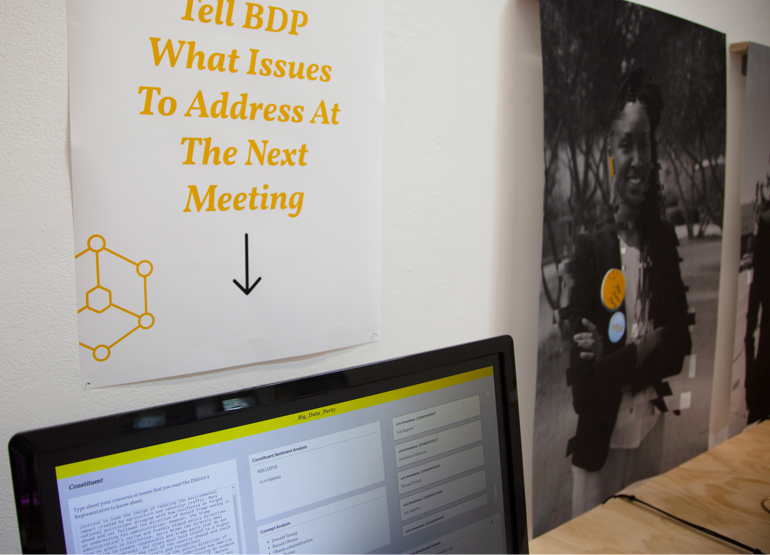
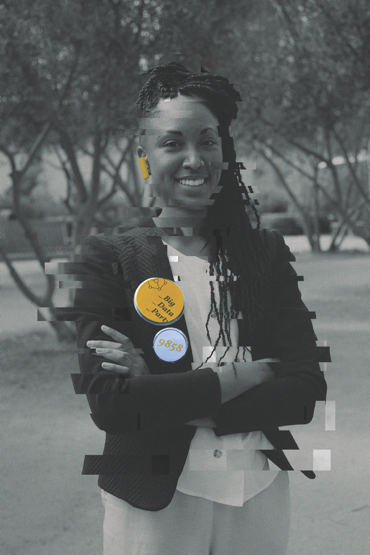
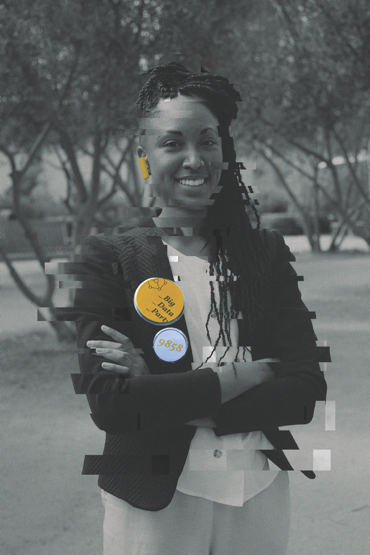
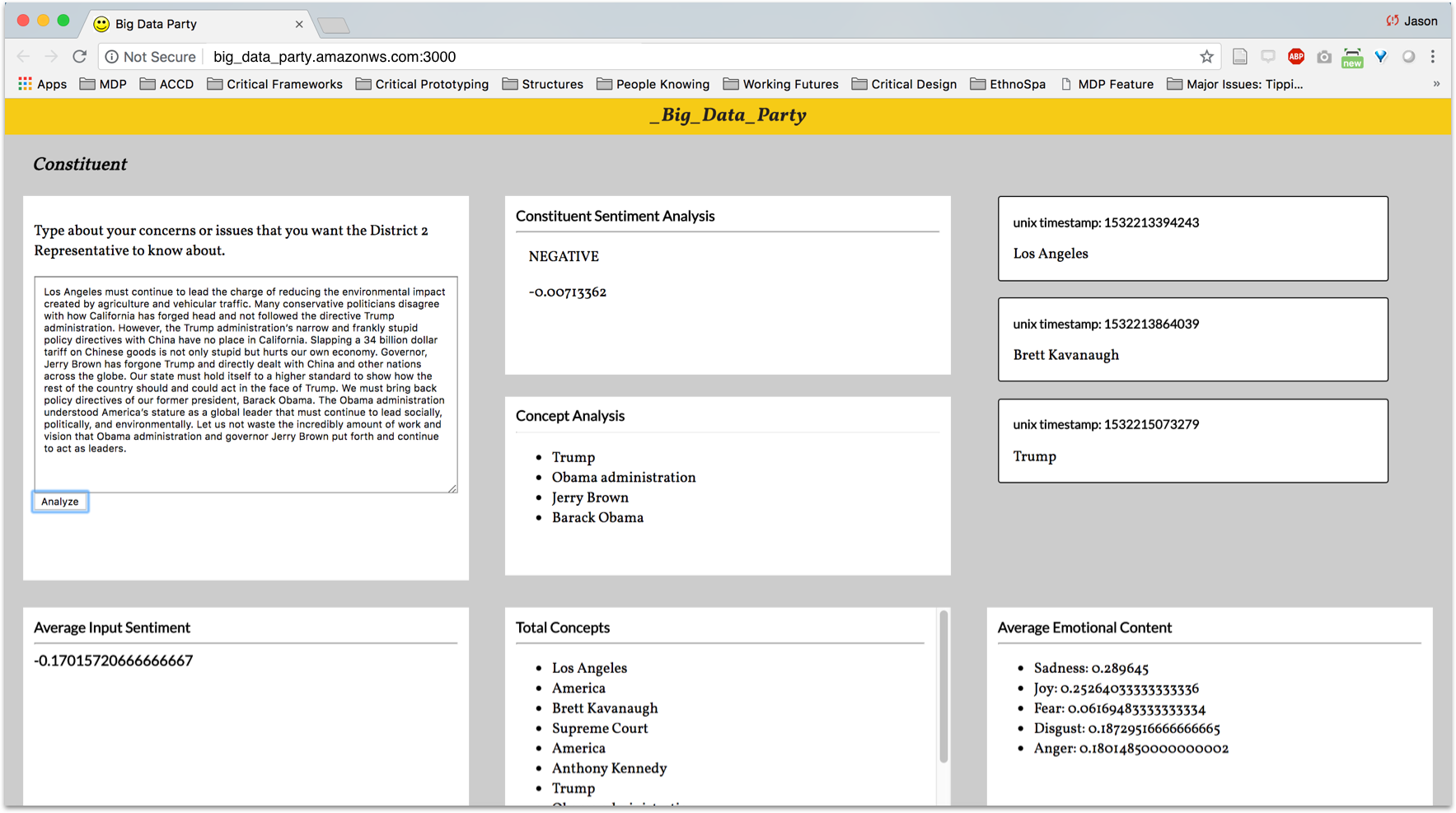
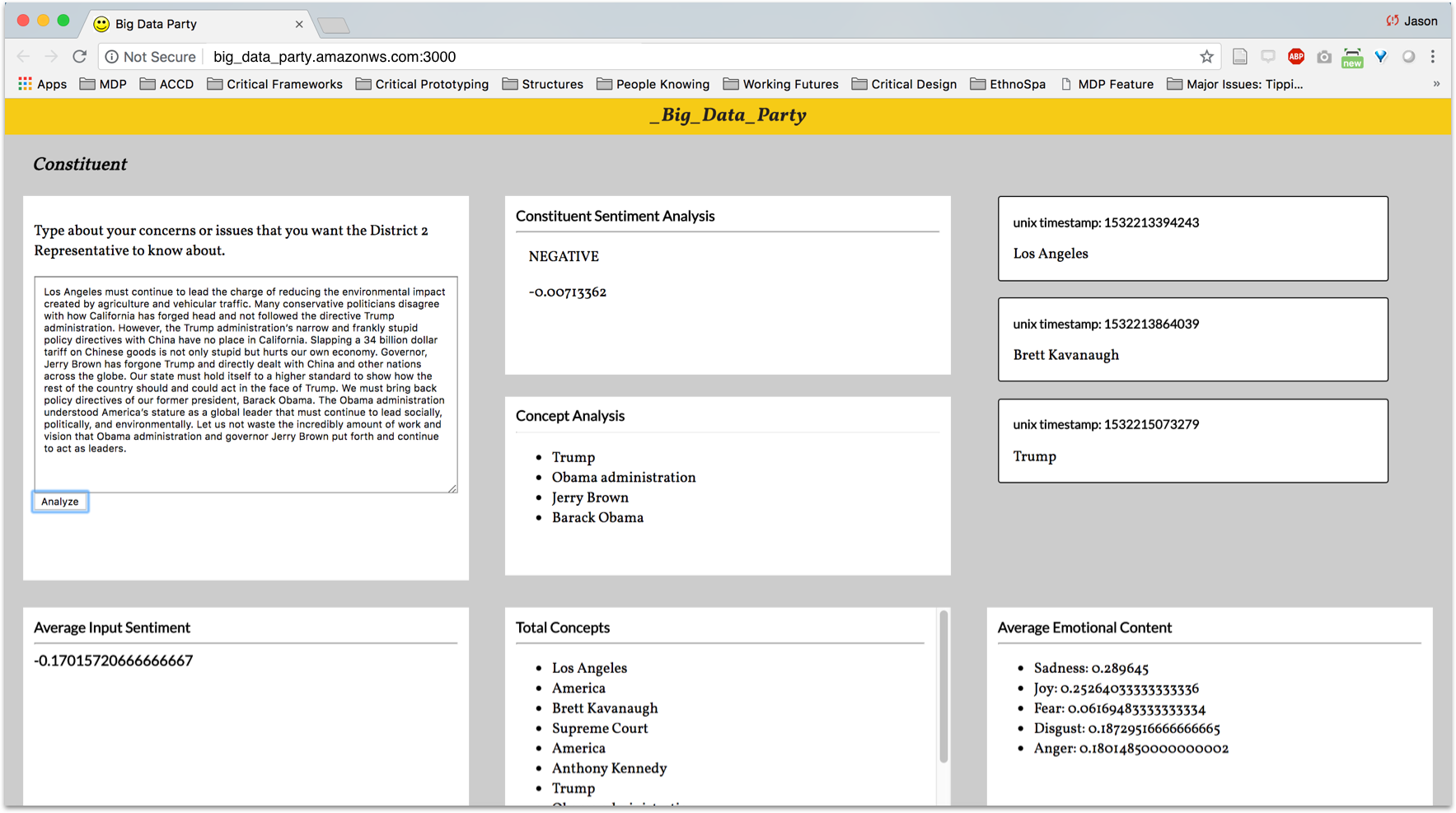
Figure 3: A collection of images depicting the installation, the _Big_Data_Party human representative, and the second iteration of the constituent sentiment tool.
Reflection
There are a few different areas at which I believe that the project could continue in. The potential of machine learning and computation could allow for holistic understanding if completed in the manner that engages with constituents by augmenting representatives. In no form do I believe that this should work as a replacement for the incredibly difficult work of local city council member, but could potentially help inform and provide insight. I believe there are three areas worth considering.
Data Acquisition and Sourcing
Create guidelines for equitable acquisition of data one that considers missing stakeholders and considers how to engage with the stakeholders.
Incorporate User Feedback
Not only from constituents but also representatives that provides timely, accessible, and comprehensible information.
Efficacy and Usefulness
Evaluate whether this is a real benefit to all stakeholders
Notes
1. Currie, Morgan. 2017. Interview by Jason Wong, November 20, 2017.
2. Hampton, Tyron. 2017. Interview by Jason Shun Wong, January 30, 2018.
3. https://www.ibm.com/watson/services/natural-language-understanding/
© Jason Wong. All Rights Reserved.